In this tutorial, you will learn how to use Keras for feature extraction on image datasets too big to fit into memory. You’ll utilize ResNet-50 (pre-trained on ImageNet) to extract features from a large image dataset, and then use incremental learning to train a classifier on top of the extracted features.
Today is part two in our three-part series on transfer learning with Keras:
- Part 1: Transfer Learning with Keras and Deep Learning (last week’s tutorial)
- Part 2: Keras: Feature extraction on large datasets (today’s post)
- Part 3: Fine-tuning with Keras and Deep Learning (next week’s tutorial)
Last week we discussed how to perform transfer learning using Keras — inside that tutorial we focused primarily on transfer learning via feature extraction.
Using this method we were able to utilize CNNs to recognize classes it was never trained on!
The problem with that method is that it assumes that all of our extracted features can fit into memory — that may not always be the case!
For example, suppose we have a dataset of 50,000 images and wanted to utilize the ResNet-50 network for feature extraction via the final layer prior to the FC layers — that output volume would be of size 7 x 7 x 2048 = 100,352-dim.
If we had 50,000 of such 100,352-dim feature vectors (assuming 32-bit floats), then we would need a total of 40.14GB of RAM to store the entire set of feature vectors in memory!
Most people don’t have 40GB+ of RAM in their machines, so in those situations, we need to be able to perform incremental learning and train our model on incremental subsets of the data.
The rest of today’s tutorial will show you how to do exactly that.
To learn how to utilize Keras for feature extraction on large datasets, just keep reading!
Keras: Feature extraction on large datasets with Deep Learning
2020-06-04 Update: This blog post is now TensorFlow 2+ compatible!
In the first part of this tutorial, we’ll briefly discuss the concept of treating networks as feature extractors (which was covered in more detail in last week’s tutorial).
From there we’ll investigate the scenario in which your extracted feature dataset is too large to fit into memory — in those situations, we’ll need to apply incremental learning to our dataset.
Next, we’ll implement Python source code that can be used for:
- Keras feature extraction
- Followed by incremental learning on the extracted features
Let’s get started!
Networks as feature extractors
When performing deep learning feature extraction, we treat the pre-trained network as an arbitrary feature extractor, allowing the input image to propagate forward, stopping at pre-specified layer, and taking the outputs of that layer as our features.
Doing so, we can still utilize the robust, discriminative features learned by the CNN. We can also use them to recognize classes the CNN was never trained on!
An example of feature extraction via deep learning can be seen in Figure 1 at the top of this section.
Here we take the VGG16 network, allow an image to forward propagate to the final max-pooling layer (prior to the fully-connected layers), and extract the activations at that layer.
The output of the max-pooling layer has a volume shape of 7 x 7 x 512 which we flatten into a feature vector of 21,055-dim.
Given a dataset of N images, we can repeat the process of feature extraction for all images in the dataset, leaving us with a total of N x 21,055-dim feature vectors.
Given these features, we can train a “standard” machine learning model (such as Logistic Regression or Linear SVM) on these features.
Note: Feature extraction via deep learning was covered in much more detail in last week’s post — refer to it if you have any questions on how feature extraction works.
What if your extracted features are too large to fit into memory?
Feature extraction via deep learning is all fine and good…
…but what happens when your extracted features are too large to fit into memory?
Keep in mind that (most implementations of, including scikit-learn) Logistic Regression and SVMs require your entire dataset to be accessible all at once for training (i.e., the entire dataset must fit into RAM).
That’s great, but if you have 50GB, 100GB, or even 1TB of extracted features, what are you going to do?
Most people don’t have access to machines with so much memory.
So, what do you do then?
Solution: Incremental learning (i.e., “online learning”)
When your entire dataset does not fit into memory you need to perform incremental learning (sometimes called “online learning”).
Incremental learning enables you to train your model on small subsets of the data called batches.
Using incremental learning the training process becomes:
- Load a small batch of data from the dataset
- Train the model on the batch
- Repeat looping through the dataset in batches, training as we go, until we reach convergence
But wait — doesn’t that process sound familiar?
It should.
It’s exactly how we train neural networks.
Neural networks are excellent examples of incremental learners.
And in fact, if you check out the scikit-learn documentation, you’ll find that the classification models for incremental learning are either NNs themselves or directly related to NNs (i.e., Perceptron
and SGDClassifier
).
Instead of using scikit-learn’s incremental learning models, we are going to implement our own neural network using Keras.
This NN will be trained on top of our extracted features from the CNN.
Our training process now becomes:
- Extract all features from our image dataset using a CNN.
- Train a simple, feedforward neural network on top of the extracted features.
Configuring your development environment
To configure your system for this tutorial, I first recommend following either of these tutorials:
Either tutorial will help you configure you system with all the necessary software for this blog post in a convenient Python virtual environment.
Please note that PyImageSearch does not recommend or support Windows for CV/DL projects.
The Food-5K dataset
The dataset we’ll be using here today is the Food-5K dataset, curated by the Multimedia Signal Processing Group (MSPG) of the Swiss Federal Institute of Technology.
This dataset consists of 5,000 images, each belonging to one of two classes:
- Food
- Non-food
Our goal today is to:
- Utilize Keras feature extraction to extract features from the Food-5K dataset using ResNet-50 pre-trained on ImageNet.
- Train a simple neural network on top of these features to recognize classes the CNN was never trained to recognize.
It’s worth noting that the entire Food-5K dataset, after feature extraction, will only occupy ~2GB of RAM if loaded all at once — that’s not the point.
The point of today’s post is to show you how to use incremental learning to train a model on the extracted features.
That way, regardless of whether you are working with 1GB of data or 100GB of data, you will know the exact steps to train a model on top of features extracted via deep learning.
Downloading the Food-5K dataset
To start, make sure you grab the source code for today’s tutorial using the “Downloads” section of the blog post.
Once you’ve downloaded the source code, change directory into transfer-learning-keras
:
$ unzip keras-feature-extraction.zip $ cd keras-feature-extraction
In my experience, I’ve found that downloading the Food-5K dataset to be a bit unreliable.
Therefore, I’ve updated this tutorial with a link to the downloadable Food-5K dataset hosted by me. Use the following link to download the dataset reliably:
Once the dataset is downloaded, go ahead and unzip it into the project folder:
$ unzip Food-5k.zip
Project structure
Go ahead and navigate back to the root directory:
$ cd ..
From there, we’re able to analyze our project structure with the tree
command:
$ tree --dirsfirst --filelimit 10 . ├── Food-5K │ ├── evaluation [1000 entries] │ ├── training [3000 entries] │ └── validation [1000 entries] ├── dataset ├── output ├── pyimagesearch │ ├── __init__.py │ └── config.py ├── build_dataset.py ├── extract_features.py ├── Food-5K.zip └── train.py 8 directories, 6 files
The config.py
file contains our configuration settings in Python form. Our other Python scripts will take advantage of the config.
Using our build_dataset.py
script, we’ll organize and output the contents of the Food-5K/
directory to the dataset folder.
From there, the extract_features.py
script will use transfer learning via feature extraction to compute feature vectors for each image. These features will be output to a CSV file.
Both build_dataset.py
and extract_features.py
were reviewed in detail last week; however, we’ll briefly walk through them again today.
Finally, we’ll review train.py
. In this Python script, we will use incremental learning to train a simple neural network on the extracted features. This script is different than last week’s tutorial and we will focus our energy here.
Our configuration file
Let’s get started by reviewing our config.py
file where we’ll store our configurations, namely the paths to our input dataset of images along with our output paths of extracted features.
Open up the config.py
file and insert the following code:
# import the necessary packages import os # initialize the path to the *original* input directory of images ORIG_INPUT_DATASET = "Food-5K" # initialize the base path to the *new* directory that will contain # our images after computing the training and testing split BASE_PATH = "dataset" # define the names of the training, testing, and validation # directories TRAIN = "training" TEST = "evaluation" VAL = "validation" # initialize the list of class label names CLASSES = ["non_food", "food"] # set the batch size BATCH_SIZE = 32 # initialize the label encoder file path and the output directory to # where the extracted features (in CSV file format) will be stored LE_PATH = os.path.sep.join(["output", "le.cpickle"]) BASE_CSV_PATH = "output"
Take the time to read through the config.py
script paying attention to the comments.
Most of the settings are related to directory and file paths which are used in the rest of our scripts.
For a full review of the configuration, be sure to refer to last week’s post.
Building the image dataset
Whenever I’m performing machine learning on a dataset (and especially Keras/deep learning), I prefer to have my dataset in the format of:
dataset_name/class_label/example_of_class_label.jpg
Maintaining this directory structure not only keeps our dataset organized on disk but also enables us to utilize Keras’ flow_from_directory
function when we get to fine-tuning later in this series of tutorials.
Since the Food-5K dataset provides pre-supplied data splits our final directory structure will have the form:
dataset_name/split_name/class_label/example_of_class_label.jpg
Again, this step isn’t always necessary, but it is a best practice (in my opinion), and one that I suggest you do as well.
At the very least it will give you experience writing Python code to organize images on disk.
Let’s use the build_dataset.py
file to build our directory structure now:
# import the necessary packages from pyimagesearch import config from imutils import paths import shutil import os # loop over the data splits for split in (config.TRAIN, config.TEST, config.VAL): # grab all image paths in the current split print("[INFO] processing '{} split'...".format(split)) p = os.path.sep.join([config.ORIG_INPUT_DATASET, split]) imagePaths = list(paths.list_images(p)) # loop over the image paths for imagePath in imagePaths: # extract class label from the filename filename = imagePath.split(os.path.sep)[-1] label = config.CLASSES[int(filename.split("_")[0])] # construct the path to the output directory dirPath = os.path.sep.join([config.BASE_PATH, split, label]) # if the output directory does not exist, create it if not os.path.exists(dirPath): os.makedirs(dirPath) # construct the path to the output image file and copy it p = os.path.sep.join([dirPath, filename]) shutil.copy2(imagePath, p)
After importing our packages on Lines 2-5, we proceed to loop over the training, testing, and validation splits (Line 8).
We create our split + class label directory structure (detailed above) and then populate the directories with the Food-5K images. The result is organized data which we can use for extracting features.
Let’s execute the script and review our directory structure once more.
You can use the “Downloads” section of this tutorial to download the source code — from there, open up a terminal and execute the following command:
$ python build_dataset.py [INFO] processing 'training split'... [INFO] processing 'evaluation split'... [INFO] processing 'validation split'...
After doing so, you will encounter the following directory structure:
$ tree --dirsfirst --filelimit 10 . ├── Food-5K │ ├── evaluation [1000 entries] │ ├── training [3000 entries] │ ├── validation [1000 entries] │ └── Food-5K.zip ├── dataset │ ├── evaluation │ │ ├── food [500 entries] │ │ └── non_food [500 entries] │ ├── training │ │ ├── food [1500 entries] │ │ └── non_food [1500 entries] │ └── validation │ ├── food [500 entries] │ └── non_food [500 entries] ├── output ├── pyimagesearch │ ├── __init__.py │ └── config.py ├── build_dataset.py ├── extract_features.py └── train.py 16 directories, 6 files
Notice that our dataset/ directory is now populated. Each subdirectory then has the following format:
split_name/class_label
With our data organized, we’re ready to move on to feature extraction.
Using Keras for deep learning feature extraction
Now that we’ve built our dataset directory structure for the project, we can:
- Use Keras to extract features via deep learning from each image in the dataset.
- Write the class labels + extracted features to disk in CSV format.
To accomplish these tasks we’ll need to implement the extract_features.py
file.
This file was covered in detail in last week’s post so we’ll only briefly review the script here as a matter of completeness:
# import the necessary packages from sklearn.preprocessing import LabelEncoder from tensorflow.keras.applications import ResNet50 from tensorflow.keras.applications.resnet50 import preprocess_input from tensorflow.keras.preprocessing.image import img_to_array from tensorflow.keras.preprocessing.image import load_img from pyimagesearch import config from imutils import paths import numpy as np import pickle import random import os # load the ResNet50 network and initialize the label encoder print("[INFO] loading network...") model = ResNet50(weights="imagenet", include_top=False) le = None # loop over the data splits for split in (config.TRAIN, config.TEST, config.VAL): # grab all image paths in the current split print("[INFO] processing '{} split'...".format(split)) p = os.path.sep.join([config.BASE_PATH, split]) imagePaths = list(paths.list_images(p)) # randomly shuffle the image paths and then extract the class # labels from the file paths random.shuffle(imagePaths) labels = [p.split(os.path.sep)[-2] for p in imagePaths] # if the label encoder is None, create it if le is None: le = LabelEncoder() le.fit(labels) # open the output CSV file for writing csvPath = os.path.sep.join([config.BASE_CSV_PATH, "{}.csv".format(split)]) csv = open(csvPath, "w")
On Line 16, ResNet is loaded while excluding the head. Pre-trained ImageNet weights are loaded into the network as well. Feature extraction via transfer learning is now possible using this pre-trained, headless network.
From there, we proceed to loop over the data splits on Line 20.
Inside, we grab all imagePaths
for the particular split
and fit our label encoder (Lines 23-34).
A CSV file is opened for writing (Lines 37-39) so that we can write our class labels and extracted features to disk.
Now that our initializations are all set, we can start looping over images in batches:
# loop over the images in batches for (b, i) in enumerate(range(0, len(imagePaths), config.BATCH_SIZE)): # extract the batch of images and labels, then initialize the # list of actual images that will be passed through the network # for feature extraction print("[INFO] processing batch {}/{}".format(b + 1, int(np.ceil(len(imagePaths) / float(config.BATCH_SIZE))))) batchPaths = imagePaths[i:i + config.BATCH_SIZE] batchLabels = le.transform(labels[i:i + config.BATCH_SIZE]) batchImages = [] # loop over the images and labels in the current batch for imagePath in batchPaths: # load the input image using the Keras helper utility # while ensuring the image is resized to 224x224 pixels image = load_img(imagePath, target_size=(224, 224)) image = img_to_array(image) # preprocess the image by (1) expanding the dimensions and # (2) subtracting the mean RGB pixel intensity from the # ImageNet dataset image = np.expand_dims(image, axis=0) image = preprocess_input(image) # add the image to the batch batchImages.append(image)
Each image
in the batch is loaded and preprocessed. From there it is appended to batchImages
.
We’ll now send the batch through ResNet to extract features:
# pass the images through the network and use the outputs as # our actual features, then reshape the features into a # flattened volume batchImages = np.vstack(batchImages) features = model.predict(batchImages, batch_size=config.BATCH_SIZE) features = features.reshape((features.shape[0], 7 * 7 * 2048)) # loop over the class labels and extracted features for (label, vec) in zip(batchLabels, features): # construct a row that exists of the class label and # extracted features vec = ",".join([str(v) for v in vec]) csv.write("{},{}\n".format(label, vec)) # close the CSV file csv.close() # serialize the label encoder to disk f = open(config.LE_PATH, "wb") f.write(pickle.dumps(le)) f.close()
Feature extraction for the batch takes place on Line 72. Using ResNet, our output layer has a volume size of 7 x 7 x 2,048. Treating the output as a feature vector, we simply flatten it into a list of 7 x 7 x 2,048 = 100,352-dim (Line 73).
The batch of feature vectors is then output to a CSV file with the first entry of each row being the class label
and the rest of the values making up the feature vec
.
We’ll repeat this process for all batches inside each split until we finish. Finally, our label encoder is dumped to disk.
For a more detailed, line-by-line review, refer to last week’s tutorial.
To extract features from our dataset, make sure you use the “Downloads” section of the guide to download the source code to this post.
From there, open up a terminal and execute the following command:
$ python extract_features.py [INFO] loading network... [INFO] processing 'training split'... ... [INFO] processing batch 92/94 [INFO] processing batch 93/94 [INFO] processing batch 94/94 [INFO] processing 'evaluation split'... ... [INFO] processing batch 30/32 [INFO] processing batch 31/32 [INFO] processing batch 32/32 [INFO] processing 'validation split'... ... [INFO] processing batch 30/32 [INFO] processing batch 31/32 [INFO] processing batch 32/32
On an NVIDIA K80 GPU the entire feature extraction process took 5m11s.
You can also run extract_features.py
on a CPU but it will take much longer.
After feature extraction is complete, you should have three CSV files in your output directory, one for each of our data splits, respectively:
$ ls -l output/ total 2655188 -rw-rw-r-- 1 ubuntu ubuntu 502570423 May 13 17:17 evaluation.csv -rw-rw-r-- 1 ubuntu ubuntu 1508474926 May 13 17:16 training.csv -rw-rw-r-- 1 ubuntu ubuntu 502285852 May 13 17:18 validation.csv
Implementing the incremental learning training script
Finally, we are now ready to utilize incremental learning to apply transfer learning via feature extraction on large datasets.
The Python script we’re implementing in this section will be responsible for:
- Constructing the simple feedforward NN architecture.
- Implementing a CSV data generator used to yield batches of labels + feature vectors to the NN.
- Training the simple NN using the data generator.
- Evaluating the feature extractor.
Open up the train.py
script and let’s get started:
# import the necessary packages from tensorflow.keras.models import Sequential from tensorflow.keras.layers import Dense from tensorflow.keras.optimizers import SGD from tensorflow.keras.utils import to_categorical from sklearn.metrics import classification_report from pyimagesearch import config import numpy as np import pickle import os
On Lines 2-10 import our required packages. Our most notable import is TensorFlow/Keras’ Sequential
API which we will use to build a simple feedforward neural network.
Several months ago I wrote a tutorial on implementing custom Keras data generators, and more specifically, yielding data from a CSV file to train a neural network with Keras.
At the time, I found that readers were a bit confused on practical applications where you would use such a generator — today is a great example of such a practical application.
Again, keep in mind that we’re assuming at the entire CSV file of extracted features will not fit into memory. Therefore, we need a custom Keras generator to yield batches of labels + data to the network so it can be trained.
Let’s implement the generator now:
def csv_feature_generator(inputPath, bs, numClasses, mode="train"): # open the input file for reading f = open(inputPath, "r") # loop indefinitely while True: # initialize our batch of data and labels data = [] labels = [] # keep looping until we reach our batch size while len(data) < bs: # attempt to read the next row of the CSV file row = f.readline()
Our csv_feature_generator
accepts four parameters:
inputPath
: The path to our input CSV file containing the extracted features.bs
: The batch size (or length) of each chunk of data.numClasses
: An integer value representing the number of classes in our data.mode
: Whether we are training or evaluating/testing.
On Line 14, we open our CSV file for reading.
Beginning on Line 17, we loop indefinitely, starting by initializing our data and labels. (Lines 19 and 20).
From there, we’ll loop until the length data
equals the batch size starting on Line 23.
We proceed by reading a line from the CSV (Line 25). Once we have the line we’ll go ahead and process it:
# check to see if the row is empty, indicating we have # reached the end of the file if row == "": # reset the file pointer to the beginning of the file # and re-read the row f.seek(0) row = f.readline() # if we are evaluating we should now break from our # loop to ensure we don't continue to fill up the # batch from samples at the beginning of the file if mode == "eval": break # extract the class label and features from the row row = row.strip().split(",") label = row[0] label = to_categorical(label, num_classes=numClasses) features = np.array(row[1:], dtype="float") # update the data and label lists data.append(features) labels.append(label) # yield the batch to the calling function yield (np.array(data), np.array(labels))
If the row
is empty, we will restart at the beginning of the file (Lines 29-32). And if we are in evaluation mode, we will break
from our loop, ensuring that we don’t fill the batch from the start of the file (Lines 38 and 39).
Assuming we are continuing on, the label
and features
are extracted from the row
(Lines 42-45).
We then append the feature vector (features
) and label
to the data
and labels
lists, respectively, until the lists reach the specified batch size (Lines 48 and 49).
When the batch is ready, Line 52 yields the data
and labels
as a tuple. Python’s yield
keyword is critical to making our function operate as a generator.
Let’s continue — we have a few more steps before we will train the model:
# load the label encoder from disk le = pickle.loads(open(config.LE_PATH, "rb").read()) # derive the paths to the training, validation, and testing CSV files trainPath = os.path.sep.join([config.BASE_CSV_PATH, "{}.csv".format(config.TRAIN)]) valPath = os.path.sep.join([config.BASE_CSV_PATH, "{}.csv".format(config.VAL)]) testPath = os.path.sep.join([config.BASE_CSV_PATH, "{}.csv".format(config.TEST)]) # determine the total number of images in the training and validation # sets totalTrain = sum([1 for l in open(trainPath)]) totalVal = sum([1 for l in open(valPath)]) # extract the testing labels from the CSV file and then determine the # number of testing images testLabels = [int(row.split(",")[0]) for row in open(testPath)] totalTest = len(testLabels)
Our label encoder is loaded from disk on Line 54. We then derive the paths to the training, validation, and testing CSV files (Lines 58-63).
Lines 67 and 68 handle counting the number of images that are in the training and validation sets. With this information, we will be able to tell the .fit_generator
function how many batch_size
steps are in each epoch.
Let’s construct a generator for each data split:
# construct the training, validation, and testing generators trainGen = csv_feature_generator(trainPath, config.BATCH_SIZE, len(config.CLASSES), mode="train") valGen = csv_feature_generator(valPath, config.BATCH_SIZE, len(config.CLASSES), mode="eval") testGen = csv_feature_generator(testPath, config.BATCH_SIZE, len(config.CLASSES), mode="eval")
Lines 76-81 initialize our CSV feature generators.
We’re now ready to build a simple neural network:
# define our simple neural network model = Sequential() model.add(Dense(256, input_shape=(7 * 7 * 2048,), activation="relu")) model.add(Dense(16, activation="relu")) model.add(Dense(len(config.CLASSES), activation="softmax"))
Contrary to last week’s tutorial where we used a Logistic Regression machine learning model, today we will build a simple neural network for classification.
Lines 84-87 define a simple 100352-256-16-2
feedforward neural network architecture using Keras.
How did I come up with the values of 256
and 16
for the two hidden layers?
A good rule of thumb is to take the square root of the previous number of nodes in the layer and then find the closest power of 2.
In this case, the closest power of 2 to 100352
is 256
. The square root of 256
is then 16
, thus giving us our architecture definition.
Let’s go ahead and compile
our model
:
# compile the model opt = SGD(lr=1e-3, momentum=0.9, decay=1e-3 / 25) model.compile(loss="binary_crossentropy", optimizer=opt, metrics=["accuracy"])
We compile
our model
using stochastic gradient descent (SGD
) with an initial learning rate of 1e-3
(which will decay over 25
epochs).
We’re using "binary_crossentropy"
for our loss
function here as we only have to two classes. If you have greater than 2 classes then you should use "categorical_crossentropy"
.
With our model
compiled, now we are ready to train and evaluate:
# train the network print("[INFO] training simple network...") H = model.fit( x=trainGen, steps_per_epoch=totalTrain // config.BATCH_SIZE, validation_data=valGen, validation_steps=totalVal // config.BATCH_SIZE, epochs=25) # make predictions on the testing images, finding the index of the # label with the corresponding largest predicted probability, then # show a nicely formatted classification report print("[INFO] evaluating network...") predIdxs = model.predict(x=testGen, steps=(totalTest //config.BATCH_SIZE) + 1) predIdxs = np.argmax(predIdxs, axis=1) print(classification_report(testLabels, predIdxs, target_names=le.classes_))
2020-06-04 Update: Formerly, TensorFlow/Keras required use of a method called .fit_generator
in order to accomplish data augmentation. Now, the .fit
method can handle data augmentation as well, making for more-consistent code. This also applies to the migration from .predict_generator
to .predict
. Be sure to check out my articles about fit and fit_generator as well as data augmentation.
Lines 96-101 fit our model
using our training and validation generators (trainGen
and valGen
). Using generators with our model
allows for incremental learning.
Using incremental learning we are no longer required to have all of our data loaded into memory at one time. Instead, batches of data flow through our network making it easy to work with massive datasets.
Of course, CSV data isn’t exactly an efficient use of space, nor is it fast. Inside of Deep Learning for Computer Vision with Python, I teach how to use HDF5 for storage more efficiently.
Evaluation of the model takes place on Lines 107-109, where testGen
generates our feature vectors in batches. A classification report is then printed in the terminal (Lines 110 and 111).
Keras feature extraction results
Finally, we are ready to train our simple NN on the extracted features from ResNet!
Make sure you use the “Downloads” section of this tutorial to download the source code.
From there, open up a terminal and execute the following command:
$ python train.py Using TensorFlow backend. [INFO] training simple network... Epoch 1/25 93/93 [==============================] - 43s 462ms/step - loss: 0.0806 - accuracy: 0.9735 - val_loss: 0.0860 - val_accuracy: 0.9798 Epoch 2/25 93/93 [==============================] - 43s 461ms/step - loss: 0.0124 - accuracy: 0.9970 - val_loss: 0.0601 - val_accuracy: 0.9849 Epoch 3/25 93/93 [==============================] - 42s 451ms/step - loss: 7.9956e-04 - accuracy: 1.0000 - val_loss: 0.0636 - val_accuracy: 0.9859 Epoch 4/25 93/93 [==============================] - 42s 450ms/step - loss: 2.3326e-04 - accuracy: 1.0000 - val_loss: 0.0658 - val_accuracy: 0.9859 Epoch 5/25 93/93 [==============================] - 43s 459ms/step - loss: 1.4288e-04 - accuracy: 1.0000 - val_loss: 0.0653 - val_accuracy: 0.9859 ... Epoch 21/25 93/93 [==============================] - 42s 456ms/step - loss: 3.3550e-05 - accuracy: 1.0000 - val_loss: 0.0661 - val_accuracy: 0.9869 Epoch 22/25 93/93 [==============================] - 42s 453ms/step - loss: 3.1843e-05 - accuracy: 1.0000 - val_loss: 0.0663 - val_accuracy: 0.9869 Epoch 23/25 93/93 [==============================] - 42s 452ms/step - loss: 3.1020e-05 - accuracy: 1.0000 - val_loss: 0.0663 - val_accuracy: 0.9869 Epoch 24/25 93/93 [==============================] - 42s 452ms/step - loss: 2.9564e-05 - accuracy: 1.0000 - val_loss: 0.0664 - val_accuracy: 0.9869 Epoch 25/25 93/93 [==============================] - 42s 454ms/step - loss: 2.8628e-05 - accuracy: 1.0000 - val_loss: 0.0665 - val_accuracy: 0.9869 [INFO] evaluating network... precision recall f1-score support food 0.99 0.99 0.99 500 non_food 0.99 0.99 0.99 500 accuracy 0.99 1000 macro avg 0.99 0.99 0.99 1000 weighted avg 0.99 0.99 0.99 1000
Training on an NVIDIA K80 took approximately ~30m. You could train on a CPU as well but it will take considerably longer.
And as our output shows, we are able to obtain ~99% accuracy on the Food-5K dataset, even though ResNet-50 was never trained on food/non-food classes!
As you can see, transfer learning is a very powerful technique, enabling you to take the features extracted from CNNs and recognize classes they were not trained on.
Later in this series of tutorials on transfer learning with Keras and deep learning, I’ll be showing you how to perform fine-tuning, another transfer learning method.
Interested in learning more about online/incremental learning?
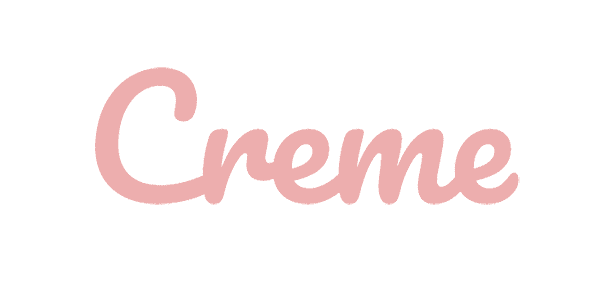
Neural networks and deep learning are a form of incremental learning — we can train such networks on one sample or one batch at a time.
However, just because we can apply neural networks to a problem doesn’t mean we should.
Instead, we need to bring the right tool to the job. Just because you have a hammer in your hand doesn’t mean you would use it to bang in a screw.
Incremental learning algorithms encompass a set of techniques used to train models in an incremental fashion.
We often utilize incremental learning when a dataset is too large to fit into memory.
The scikit-learn library does include a small handful of online learning algorithms, however:
- It does not treat incremental learning as a first-class citizen.
- The implementations are awkward to use.
Enter the Creme library — a library exclusively dedicated to incremental learning with Python.
I really enjoyed my first experience working with creme and found the scikit-learn inspired API very easy to use.
Click here to read my Online/Incremental Learning with Keras and Creme article!
What's next? We recommend PyImageSearch University.
84 total classes • 114+ hours of on-demand code walkthrough videos • Last updated: February 2024
★★★★★ 4.84 (128 Ratings) • 16,000+ Students Enrolled
I strongly believe that if you had the right teacher you could master computer vision and deep learning.
Do you think learning computer vision and deep learning has to be time-consuming, overwhelming, and complicated? Or has to involve complex mathematics and equations? Or requires a degree in computer science?
That’s not the case.
All you need to master computer vision and deep learning is for someone to explain things to you in simple, intuitive terms. And that’s exactly what I do. My mission is to change education and how complex Artificial Intelligence topics are taught.
If you're serious about learning computer vision, your next stop should be PyImageSearch University, the most comprehensive computer vision, deep learning, and OpenCV course online today. Here you’ll learn how to successfully and confidently apply computer vision to your work, research, and projects. Join me in computer vision mastery.
Inside PyImageSearch University you'll find:
- ✓ 84 courses on essential computer vision, deep learning, and OpenCV topics
- ✓ 84 Certificates of Completion
- ✓ 114+ hours of on-demand video
- ✓ Brand new courses released regularly, ensuring you can keep up with state-of-the-art techniques
- ✓ Pre-configured Jupyter Notebooks in Google Colab
- ✓ Run all code examples in your web browser — works on Windows, macOS, and Linux (no dev environment configuration required!)
- ✓ Access to centralized code repos for all 536+ tutorials on PyImageSearch
- ✓ Easy one-click downloads for code, datasets, pre-trained models, etc.
- ✓ Access on mobile, laptop, desktop, etc.
Summary
In this tutorial you learned how to:
- Utilize Keras for deep learning feature extraction.
- Perform incremental learning on the extracted features.
Utilizing incremental learning enables us to train models on datasets too large to fit into memory.
Neural networks are a great example of incremental learners as we can load data via batches, ensuring the entire network does not have to fit into RAM at once. Using incremental learning we were able to obtain ~98% accuracy.
I would suggest using this code as a template for whenever you need to use Keras for feature extraction on large datasets.
I hope you enjoyed the tutorial!
To download the source code to this post (and be notified when future tutorials are published here on PyImageSearch), just enter your email address in the form below!
Download the Source Code and FREE 17-page Resource Guide
Enter your email address below to get a .zip of the code and a FREE 17-page Resource Guide on Computer Vision, OpenCV, and Deep Learning. Inside you'll find my hand-picked tutorials, books, courses, and libraries to help you master CV and DL!